Set up human evaluation
Set up human raters to review and assess AI outputs for quality control
Human evaluation is essential for maintaining quality control and oversight of your AI system's outputs. Create structured workflows for human reviewers to rate and provide feedback on AI responses.
Add evaluation instructions
Write clear guidelines for human reviewers. These instructions appear during the review process and should include:
- What aspects to evaluate
- How to assign ratings
- Examples of good and bad responses
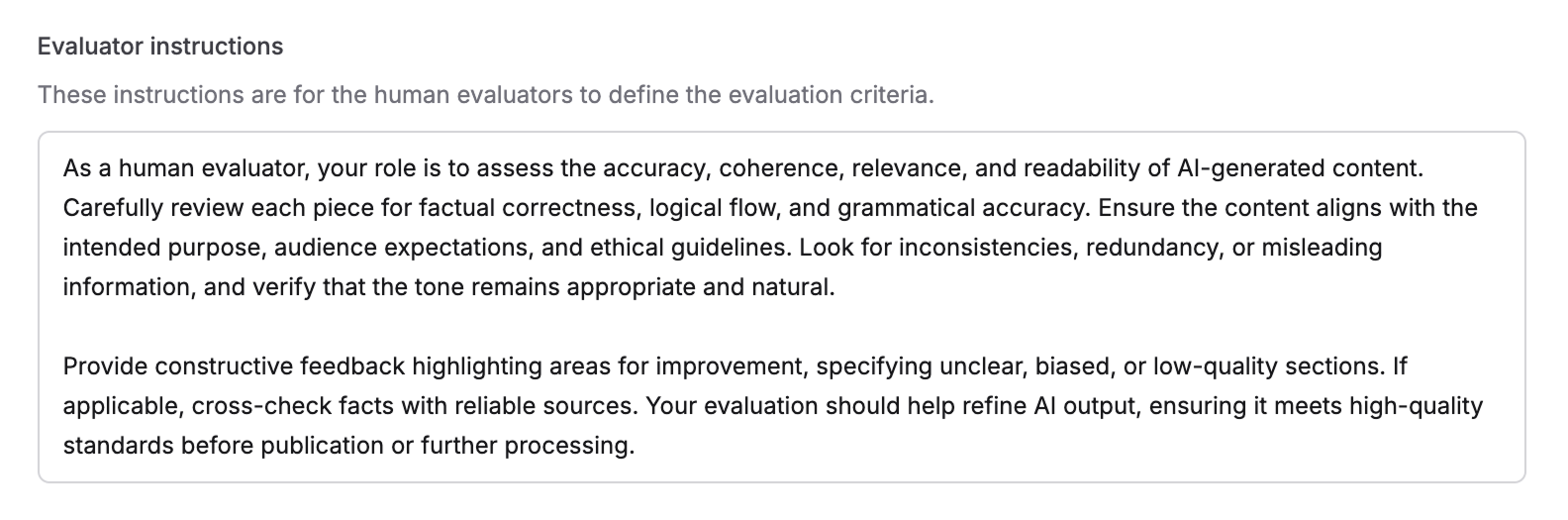
Select evaluation type
Choose between two rating formats:
Binary (Yes/No) Simple binary evaluation

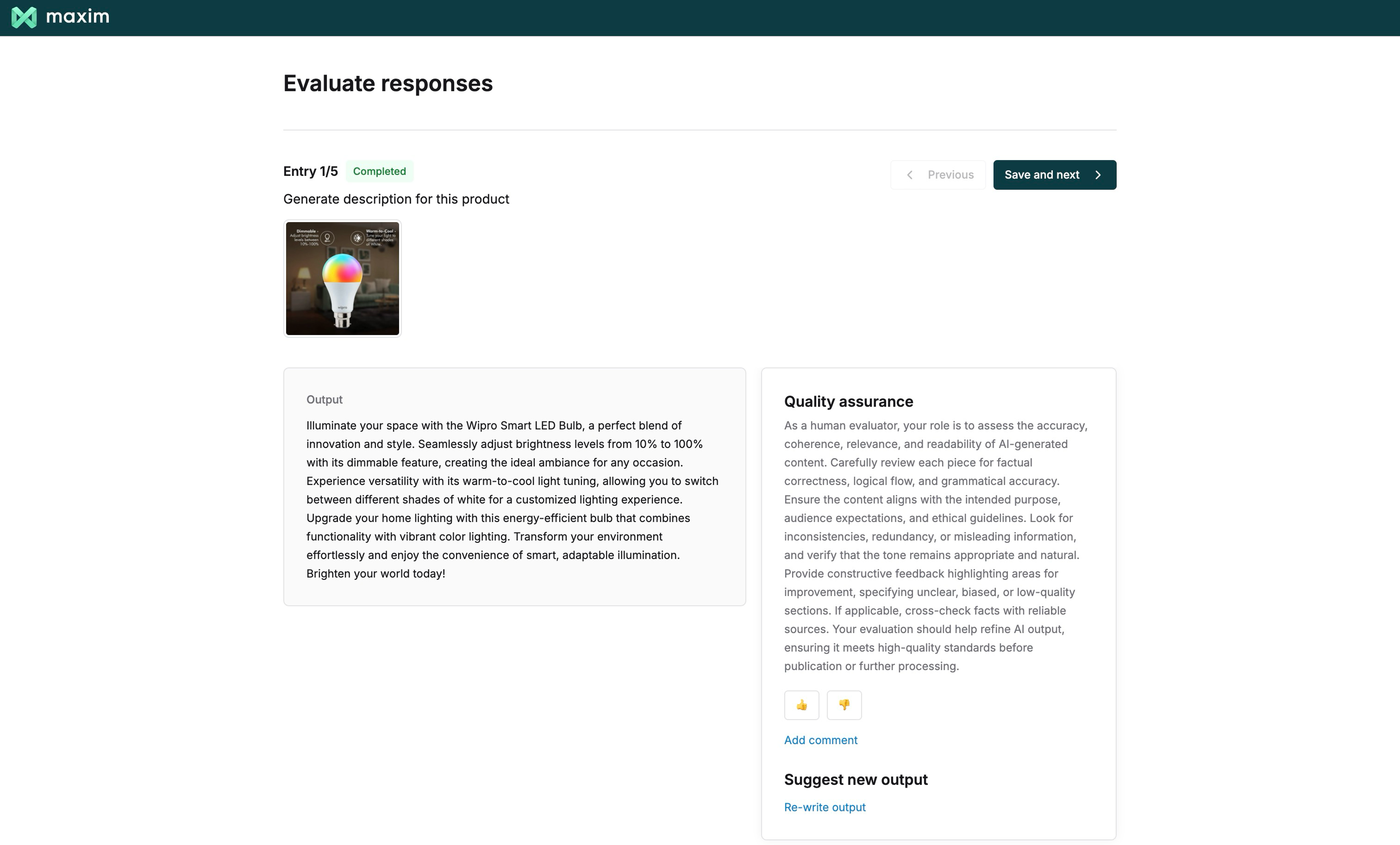
Scale Nuanced rating system for detailed quality assessment
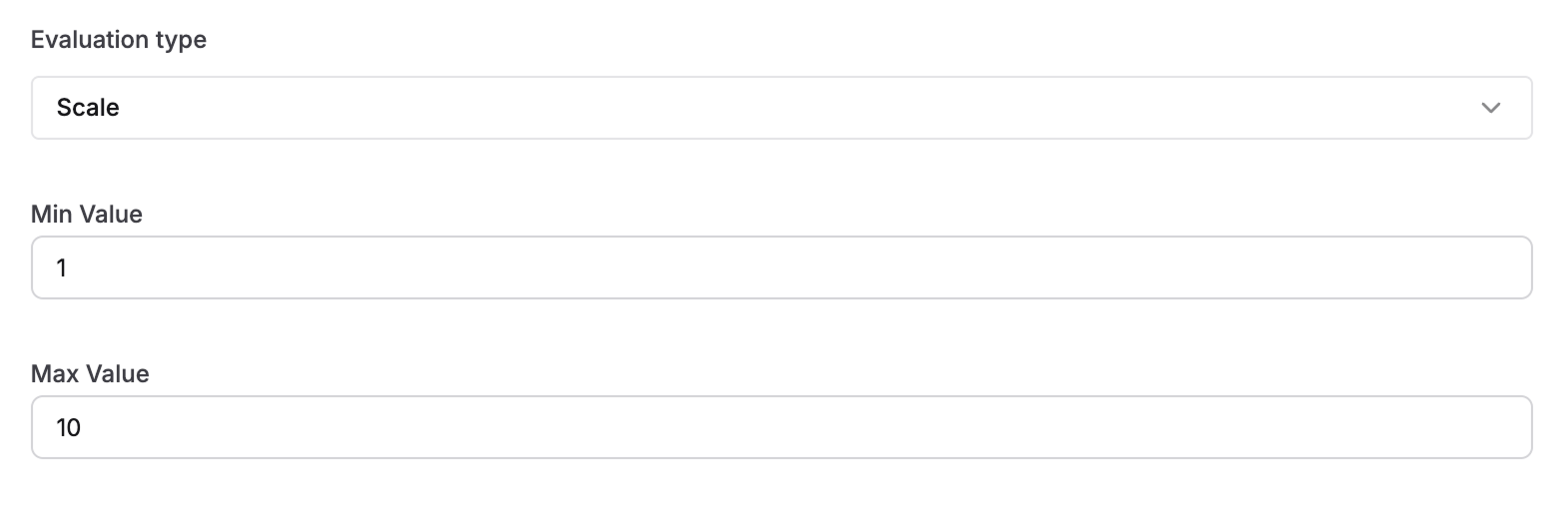
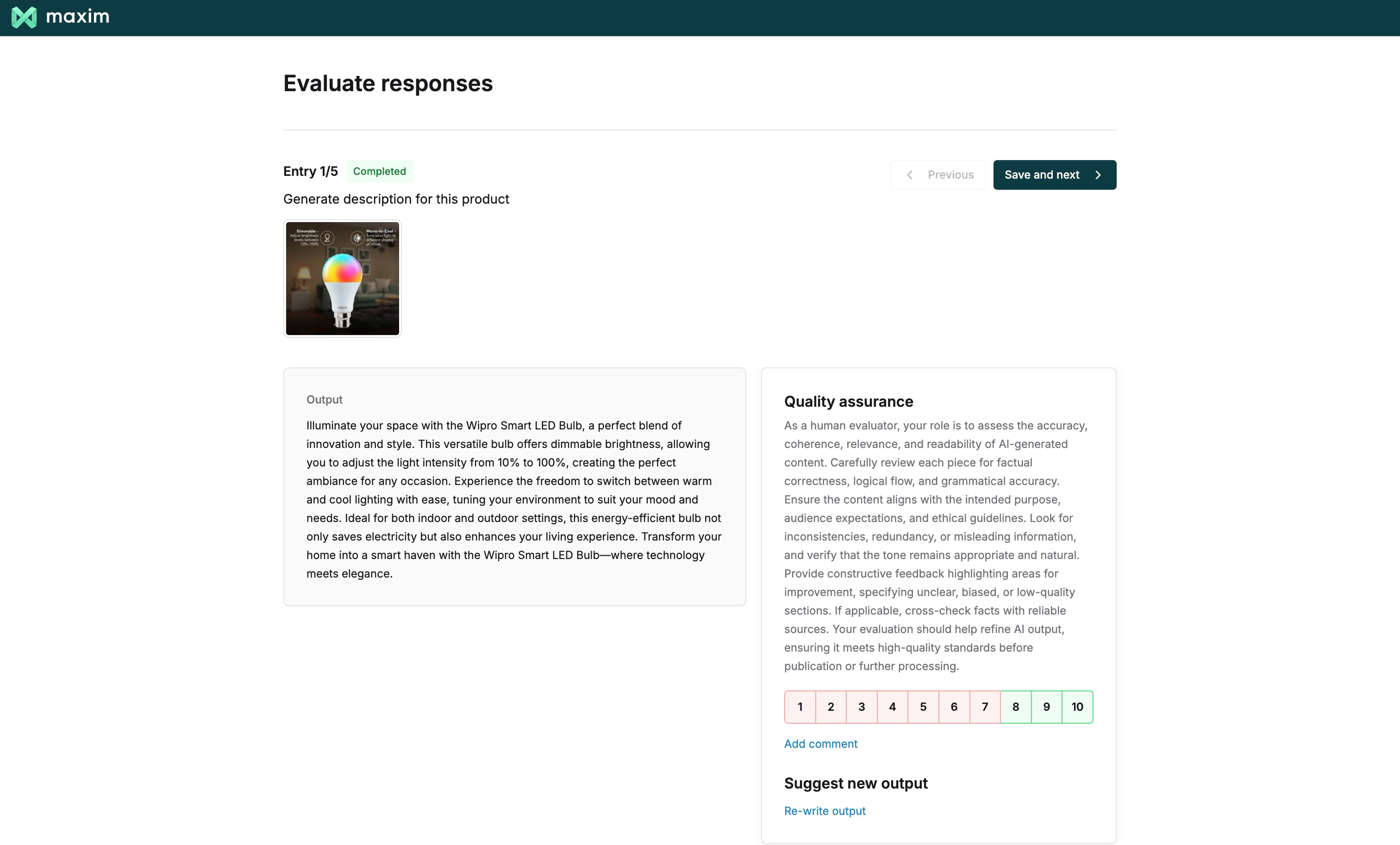
Set pass criteria
Configure two types of pass criteria:
Pass query Define criteria for individual evaluation metrics
Example: Pass if evaluation score > 0.8
Pass evaluator (%) Set threshold for overall evaluation across multiple entries
Example: Pass if 80% of entries meet the human evaluation criteria
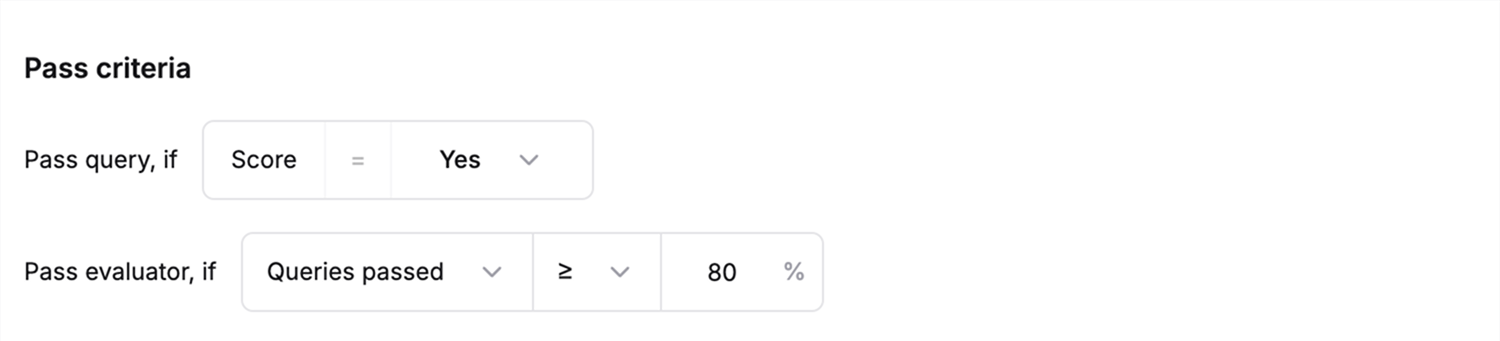